

In these cases I don't mean that they will always get good OOD accuracy. The in-distribution accuracy is high (for a binary task, something like 97% or higher).The in-distribution data is reasonably diverse.
Intuitively, neural networks "want" to generalize, and will make reasonable extrapolations as long as: The question is: how will neural networks behave in "out-of-distribution" situations where the training data hasn't fully pinned down their behavior? On a spectrum from "completely randomly" (0) to "exactly as intended" (10), my current view is around an 8/10. This one is a bit more fuzzy and qualitative, and is a prediction about the future rather than empirical evidence about the past. I also expect this to continue holding even after ML models gain some new emergent capability such as good long-term planning. The findings above are in computer vision and NLP, but I'd bet that in pretty much any domain more unsupervised data will mean you need less supervised data, and that this trend will hold until you're close to information-theoretic limits (i.e. Taken to its logical extreme, this meant that with enough data you should be able to learn from very few examples-which is what's happened, for both fine-tuning and few-shot learning. Moreover, it seemed that pre-training on more and better data increased data efficiency further. Starting around 2016, there were papers showing that learned representations from pre-trained models were more data-efficient compared to randomly-initialized models. Moreover, the main (partial) remedy, adversarial training, is the same in every architecture and domain.ĭata efficiency. As far as I know, adversarial examples affect every neural network model that exists. Since then, there have been at least two qualitative changes in deep networks-pretraining to provide better inductive bias, and the emergence of few-shot learning-plus some smaller changes in architecture.
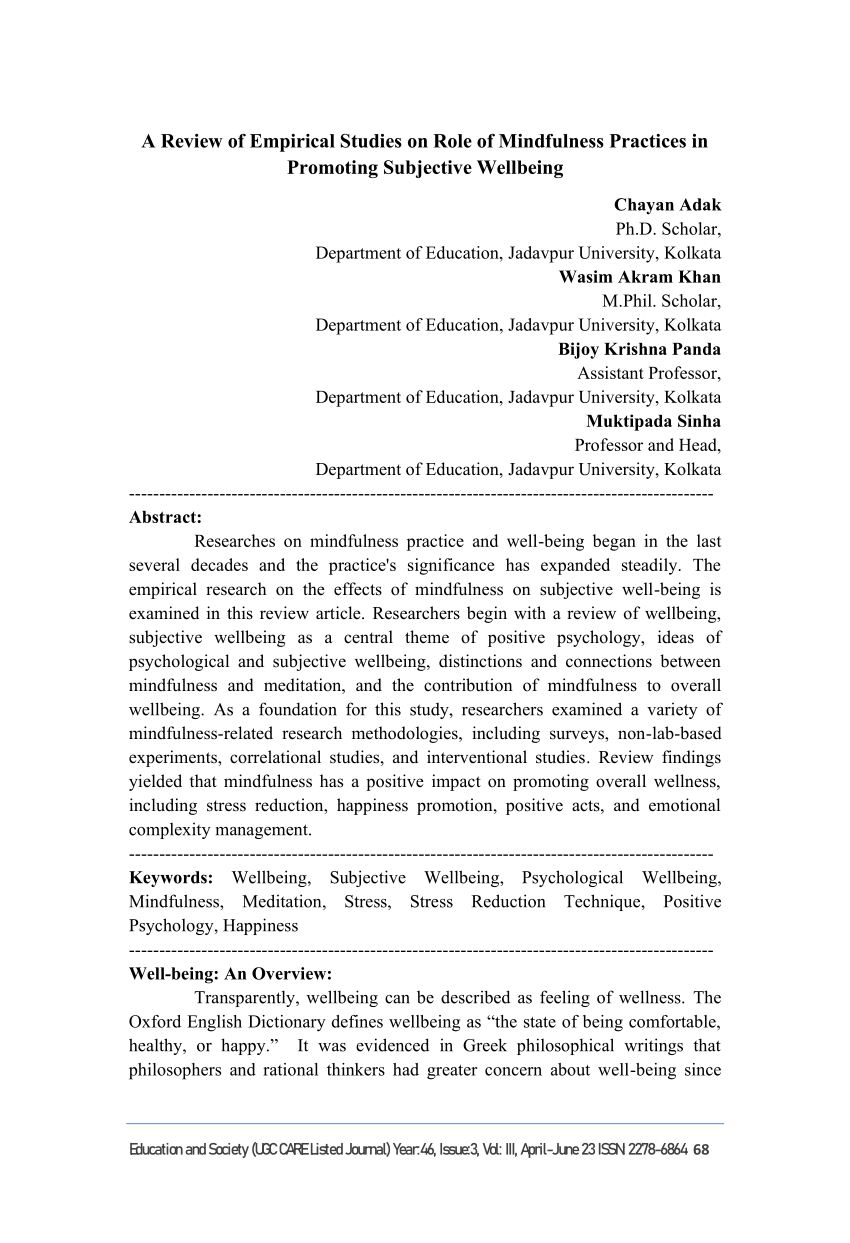
Adversarial examples were first discovered in 2013, a year after the AlexNet paper (which arguably marked the start of "modern" deep learning). I'll consider three examples in deep learning: adversarial examples, data efficiency, and out-of-distribution generalization.Īdversarial examples. Empirical Generalization in Deep Learning Since "modern" deep learning hasn't been around that long, I'll also look at examples from biology, a field that has been around for a relatively long time and where More Is Different is ubiquitous (see Appendix: More Is Different In Other Domains). So, to make my case, I'll start by considering examples in deep learning that have held up in this way. Moreover, we care specifically about findings that continue to hold up after some sort of emergent behavior (such as few-shot learning in the case of ML). However, just invoking physics isn't a good argument, because physical laws have fundamental symmetries that we shouldn't expect in machine learning. This is one of the big lessons of physics, and while some might attribute physics' success to math instead of empiricism, I think it's clear that you need empirical data to point to the right mathematics. I don't think many people would contest the claim that empirical investigation can uncover deep and generalizable truths. Findings do often generalize, but you need to think to determine the right generalization, and also about what might stop any given generalization from holding. This might seem like a contradiction, but actually I think divergence from current trends and empirical generalization are consistent. In this post, I will argue that despite this, empirical findings often do generalize very far, including across "phase transitions" caused by emergent behavior. Previously, I argued that emergent phenomena in machine learning mean that we can't rely on current trends to predict what the future of ML will be like.
